THE CHALLENGE
Detailed analysis of costs and benefits
A lot of bad decisions were made because of poor cost and profit analysis. A company wants to have a flexible and business-friendly tool for a detailed analysis of costs and profits for making the right business decisions fast.Estimation of Bottlenecks and Peaks
Not properly planned business bottlenecks and peak hours/days could result in losses and unsatisfied customers. The company wants to have a better and more accurate plan for resources and warehouses utilization in an advanced way.Operational capacity planning
Not properly planned business bottlenecks and peak hours/days could result in losses and unsatisfied customers. The company wants to have a better and more accurate plan for resources and warehouses utilization in an advanced way.OUR SOLUTION
Embedded analytics
After the solution deployment, the company was able to analyze large amounts of disparate data with quick response by using internal applications. The company was also able to extract disseminate business insights in real-time and share those insights by using interactive dashboards with decision-makers. Such analytic applications became a real backbone for the future of the supply chain.Machine Learning in Logistics – predictive modeling
Predictive analytics is improving the supply chain and logistics industry by being able to accurately collect and analyze data that helps in making management decisions. It can also help address issues like damaged inventory, stock errors, supply and demand miscalculations. Predictive capabilities allow organizations to accurately address customer services and traffic patterns, labor unrest, and weather events that affect shipping and port behavior.Data integration (ETL)
The data integration phase involved the extraction, transformation, and data loading into one information management system while adhering to particular business rules. Data were integrated from various sources so it gave a possibility to analyze the business from various perspectives.Machine Learning in Logistics – route optimization
The created algorithm now ensures maximum utilization of assets with tight control on wastage. It helps to automate and optimize logistics routes. This results in reduced logistics cost, on-time deliveries and delightful end-user experience.BENEFITS
The solution provides the organization with a full range of insights and information about the organization’s condition. It also helps to make the right critical decisions.
34%
Increased operational efficiency
1
Master data source
20.000$
in savings
TECHNOLOGIES USED
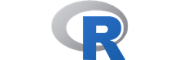
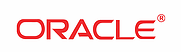
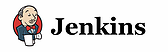
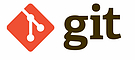